Catalogue Search | MBRL
نتائج البحث
MBRLSearchResults
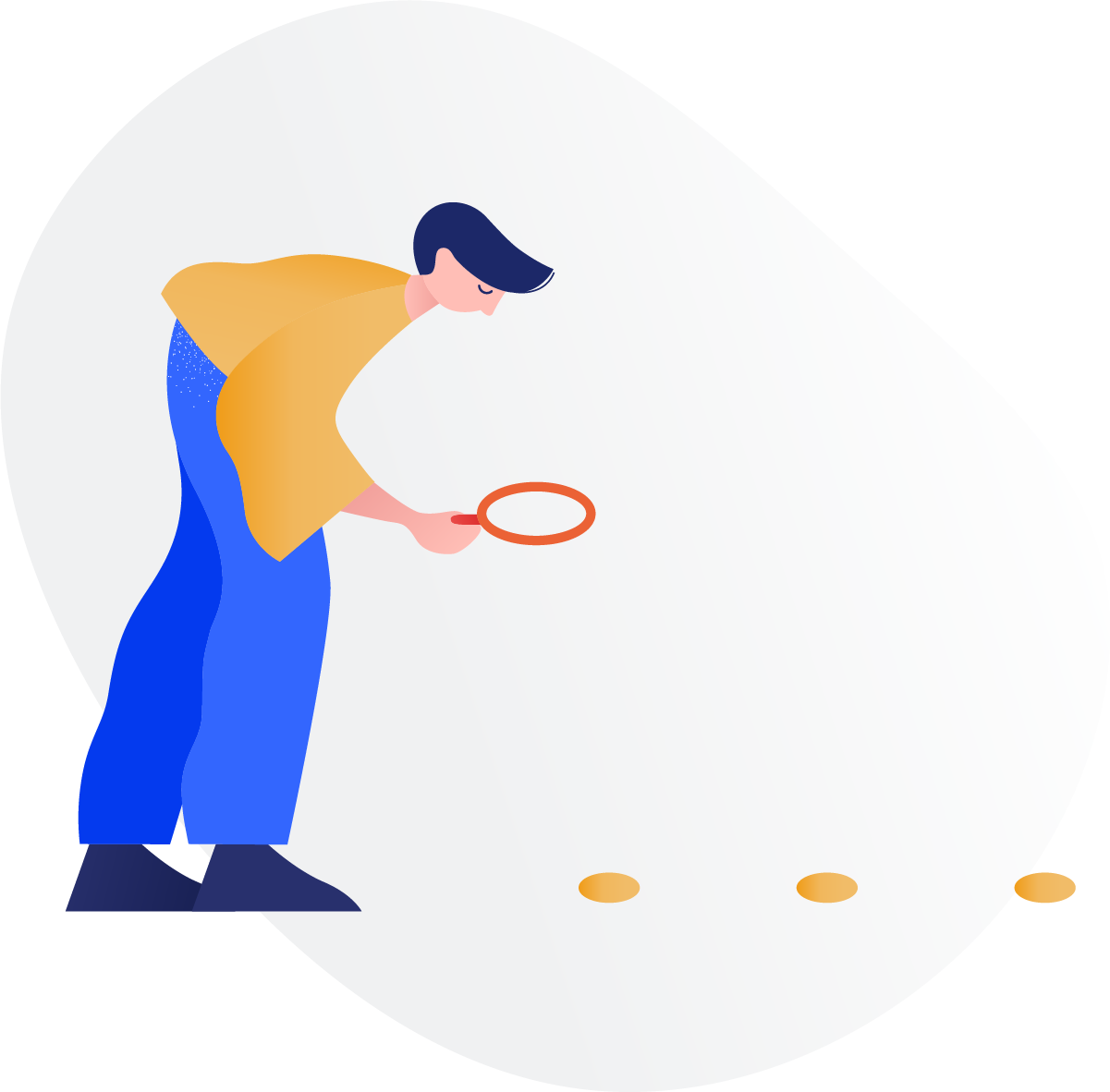
وجه الفتاة! هناك خطأ ما.
أثناء محاولة إضافة العنوان إلى الرف ، حدث خطأ ما :( يرجى إعادة المحاولة لاحقًا!
-
الضبطالضبط
-
مُحَكَّمةمُحَكَّمة
-
نوع العنصرنوع العنصر
-
الموضوعالموضوع
-
السنةمن:-إلى:
-
المزيد من المرشحاتالمزيد من المرشحاتالمصدراللغة
منجز
مرشحات
إعادة تعيين
122,917
نتائج ل
"Skin cancer"
صنف حسب:
Updates on the Management of Non-Melanoma Skin Cancer (NMSC)
بواسطة
Wong, Alex K
,
Wolfswinkel, Erik M
,
Fahradyan, Artur
في
basal cell carcinoma
,
cutaneous squamous cell carcinoma
,
Health care
2017
Non-melanoma skin cancers (NMSCs) are the most common malignancy worldwide, of which 99% are basal cell carcinomas (BCCs) and squamous cell carcinomas (SCCs) of skin. NMSCs are generally considered a curable diseases, yet they currently pose an increasing global healthcare problem due to rising incidence. This has led to a shift in emphasis on prevention of NMSCs with development of various skin cancer prevention programs worldwide. This article aims to summarize the most recent changes and advances made in NMSC management with a focus on prevention, screening, diagnosis, and staging.
Journal Article
The impact of patient clinical information on automated skin cancer detection
2020
Skin cancer is one of the most common types of cancer worldwide. Over the past few years, different approaches have been proposed to deal with automated skin cancer detection. Nonetheless, most of them are based only on dermoscopic images and do not take into account the patient clinical information, an important clue towards clinical diagnosis. In this work, we present an approach to fill this gap. First, we introduce a new dataset composed of clinical images, collected using smartphones, and clinical data related to the patient. Next, we propose a straightforward method that includes an aggregation mechanism in well-known deep learning models to combine features from images and clinical data. Last, we carry out experiments to compare the models’ performance with and without using this mechanism. The results present an improvement of approximately 7% in balanced accuracy when the aggregation method is applied. Overall, the impact of clinical data on models’ performance is significant and shows the importance of including these features on automated skin cancer detection.
•A new skin cancer dataset containing clinical data and images is presented.•A straightforward approach to combine both types of data is proposed.•Well-known deep learning models are applied to the presented dataset.•The results considering clinical data and images present a notable improvement.
Journal Article
Photodynamic Therapy for Non-Melanoma Skin Cancers
2016
Non-melanoma skin cancer (NMSC) is traditionally treated with surgical excision. Nonsurgical methods such as cryotherapy and topical chemotherapeutics, amongst other treatments, are other options. Actinic keratosis (AKs) are considered precancerous lesions that eventually may progress to squamous cell carcinoma (SCC). Photodynamic therapy (PDT) offers an effective treatment for AKs, and is also effective for superficial basal cell carcinoma (BCC). Nodular BCC and Bowen's disease (SCC in situ) have shown acceptable response rates with PDT, although recurrence rates are higher for these two NMSC subtypes. Methylaminolevulinate (MAL) PDT is a more effective treatment option than 5-aminolevulinic acid (ALA) PDT for nodular BCC. Several studies have shown that PDT results in superior cosmetic outcomes compared to surgical treatment. PDT is overall well-tolerated, with pain being the most common side effect.
Journal Article
Tissue-specific opposing functions of the inflammasome adaptor ASC in the regulation of epithelial skin carcinogenesis
بواسطة
Drexler, Stefan K
,
Yazdi, Amir S
,
Masin, Mark
في
9,10-Dimethyl-1,2-benzanthracene
,
Adaptor Proteins, Signal Transducing - metabolism
,
Animals
2012
A chronic inflammatory microenvironment favors tumor progression through molecular mechanisms that are still incompletely defined. In inflammation-induced skin cancers, IL-1 receptor- or caspase-1–deficient mice, or mice specifically deficient for the inflammasome adaptor protein ASC (apoptosis-associated speck-like protein containing a CARD) in myeloid cells, had reduced tumor incidence, pointing to a role for IL-1 signaling and inflammasome activation in tumor development. However, mice fully deficient for ASC were not protected, and mice specifically deficient for ASC in keratinocytes developed more tumors than controls, suggesting that, in contrast to its proinflammatory role in myeloid cells, ASC acts as a tumor-suppressor in keratinocytes. Accordingly, ASC protein expression was lost in human cutaneous squamous cell carcinoma, but not in psoriatic skin lesions. Stimulation of primary mouse keratinocytes or the human keratinocyte cell line HaCaT with UVB induced an ASC-dependent phosphorylation of p53 and expression of p53 target genes. In HaCaT cells, ASC interacted with p53 at the endogenous level upon UVB irradiation. Thus, ASC in different tissues may influence tumor growth in opposite directions: it has a proinflammatory role in infiltrating cells that favors tumor development, but it also limits keratinocyte proliferation in response to noxious stimuli, possibly through p53 activation, which helps suppressing tumors.
Journal Article
Dermatoscopy of Neoplastic Skin Lesions: Recent Advances, Updates, and Revisions
2018
Opinion statement
Dermatoscopy (dermoscopy) improves the diagnosis of benign and malignant cutaneous neoplasms in comparison with examination with the unaided eye and should be used routinely for all pigmented and non-pigmented cutaneous neoplasms. It is especially useful for the early stage of melanoma when melanoma-specific criteria are invisible to the unaided eye. Preselection by the unaided eye is therefore not recommended. The increased availability of polarized dermatoscopes, and the extended use of dermatoscopy in non-pigmented lesions led to the discovery of new criteria, and we recommend that lesions should be examined with polarized and non-polarized dermatoscopy. The “chaos and clues algorithm” is a good starting point for beginners because it is easy to use, accurate, and it works for all types of pigmented lesions not only for those melanocytic. Physicians, who use dermatoscopy routinely, should be aware of new clues for acral melanomas, nail matrix melanomas, melanoma in situ, and nodular melanoma. Dermatoscopy should also be used to distinguish between different subtypes of basal cell carcinoma and to discriminate highly from poorly differentiated squamous cell carcinomas to optimize therapy and management of non-melanoma skin cancer. One of the most exciting areas of research is the use of dermatoscopic images for machine learning and automated diagnosis. Convolutional neural networks trained with dermatoscopic images are able to diagnose pigmented lesions with the same accuracy as human experts. We humans should not be afraid of this new and exciting development because it will most likely lead to a peaceful and fruitful coexistence of human experts and decision support systems.
Journal Article
Melanoma Classification Using a Novel Deep Convolutional Neural Network with Dermoscopic Images
بواسطة
Lindén, Maria
,
Sinha, Roopak
,
Kaur, Ranpreet
في
Artificial intelligence
,
Classification
,
Classification (of information)
2022
Automatic melanoma detection from dermoscopic skin samples is a very challenging task. However, using a deep learning approach as a machine vision tool can overcome some challenges. This research proposes an automated melanoma classifier based on a deep convolutional neural network (DCNN) to accurately classify malignant vs. benign melanoma. The structure of the DCNN is carefully designed by organizing many layers that are responsible for extracting low to high-level features of the skin images in a unique fashion. Other vital criteria in the design of DCNN are the selection of multiple filters and their sizes, employing proper deep learning layers, choosing the depth of the network, and optimizing hyperparameters. The primary objective is to propose a lightweight and less complex DCNN than other state-of-the-art methods to classify melanoma skin cancer with high efficiency. For this study, dermoscopic images containing different cancer samples were obtained from the International Skin Imaging Collaboration datastores (ISIC 2016, ISIC2017, and ISIC 2020). We evaluated the model based on accuracy, precision, recall, specificity, and F1-score. The proposed DCNN classifier achieved accuracies of 81.41%, 88.23%, and 90.42% on the ISIC 2016, 2017, and 2020 datasets, respectively, demonstrating high performance compared with the other state-of-the-art networks. Therefore, this proposed approach could provide a less complex and advanced framework for automating the melanoma diagnostic process and expediting the identification process to save a life.
Journal Article
Beyond Nicotinamide Metabolism: Potential Role of Nicotinamide N -Methyltransferase as a Biomarker in Skin Cancers
بواسطة
Campagna, Roberto
,
Salvolini, Eleonora
,
Brisigotti, Valerio
في
Adenosine triphosphate
,
Aggressive behavior
,
biomarker
2021
Skin cancers (SC) collectively represent the most common type of malignancy in white populations. SC includes two main forms: malignant melanoma and non-melanoma skin cancer (NMSC). NMSC includes different subtypes, namely, basal cell carcinoma (BCC), squamous cell carcinoma (SCC), Merkel cell carcinoma (MCC), and keratoacanthoma (KA), together with the two pre-neoplastic conditions Bowen disease (BD) and actinic keratosis (AK). Both malignant melanoma and NMSC are showing an increasing incidence rate worldwide, thus representing an important challenge for health care systems, also because, with some exceptions, SC are generally characterized by an aggressive behavior and are often diagnosed late. Thus, identifying new biomarkers suitable for diagnosis, as well as for prognosis and targeted therapy is mandatory. Nicotinamide
-methyltransferase (NNMT) is an enzyme that is emerging as a crucial player in the progression of several malignancies, while its substrate, nicotinamide, is known to exert chemopreventive effects. Since there is increasing evidence regarding the involvement of this enzyme in the malignant behavior of SC, the current review aims to summarize the state of the art as concerns NNMT role in SC and to support future studies focused on exploring the diagnostic and prognostic potential of NNMT in skin malignancies and its suitability for targeted therapy.
Journal Article
A DE-ANN Inspired Skin Cancer Detection Approach Using Fuzzy C-Means Clustering
بواسطة
AlGhamdi, Rayed
,
Sharma, Purushottam
,
Deep, Vikas
في
Accuracy
,
Artificial neural networks
,
Cancer
2020
As per recent developments in medical science, the skin cancer is considered as one of the common type disease in human body. Although the presence of melanoma is viewed as a form of cancer, it is challenging to predict it. If melanoma or other skin diseases are identified in the early stages, prognosis can then be successfully achieved to cure them. For this, medical imaging science plays an essential role in detecting such types of skin lesions quickly and accurately. The application of our approaches is to improve skin cancer detection accuracy in medical imaging and further, can be automated using electronic devices such as mobile phones etc. In the proposed paper, an improved strategy to detect three type of skin cancers in early stages are suggested. The considered input is a skin lesion image which by using the proposed method, the system would classify it into cancerous or non-cancerous type of skin. The image segmentation is implemented using fuzzy C-means clustering to separate homogeneous image regions. The preprocessing is done using different filters to enhance the image attributes while the other features are assessed by implementing rgb color-space, Local Binary Pattern (LBP) and GLCM methods altogether. Further, for classification, artificial neural network (ANN) is trained using differential evolution (DE) algorithm. Various features are accurately estimated to achieve better results using skin cancer image datasets namely HAM10000 and PH2. The novelty of the work suggests that DE-ANN is best compared among other traditional classifiers in terms of detection accuracy as discussed in result section of this paper. The simulated result shows that the proposed technique effectually detects skin cancer and produces an accuracy of 97.4%. The results are highly accurate compare to other traditional approaches in the same domain.
Journal Article
The Human Microbiota and Skin Cancer
2022
Skin cancer is the most common type of cancer in the US with an increasing prevalence worldwide. While ultraviolet (UV) radiation is a well-known risk factor, there is emerging evidence that the microbiota may also contribute. In recent years, the human microbiota has become a topic of great interest, and its association with inflammatory skin diseases (i.e., atopic dermatitis, acne, rosacea) has been explored. Little is known of the role of microbiota in skin cancer, but with the recognized link between microbial dysbiosis and inflammation, and knowledge that microbiota modulates the effect of UV-induced immunosuppression, theories connecting the two have surfaced. In this paper, we provide a comprehensive review of the key literature on human microbiota, especially the skin microbiota, and skin cancer (i.e., non-melanoma skin cancer, melanoma, cutaneous T cell lymphoma). Also, mechanistic perspectives as to how our microbiota influence skin cancer development and treatment are offered.
Journal Article
Eye color and the risk of skin cancer
بواسطة
Li, Tricia
,
Qureshi, Abrar A.
,
Cho, Eunyoung
في
Biomedical and Life Sciences
,
Biomedicine
,
Cancer
2022
Melanoma, basal cell carcinoma (BCC) and squamous cell carcinoma (SCC) are the most common skin cancers. The incidence rates of all three types of skin cancers have increased in the past three decades. Light pigmentary traits have been recognized as one of the host risk factors for skin cancer, but findings on associations between eye colors and risk of skin cancers have been inconsistent.
We performed a prospective analysis to examine the association between eye colors and risk of skin cancers using the Health Professionals Follow-up Study (HPFS). Cox proportional hazard models were applied to estimate relative risks (RRs) and their 95% confidence intervals (CIs). Effect modifications due to hair color and skin reaction to sun were also examined.
The HPFS included 35,662 males. During a median follow-up of 19 years (1988–2012), 445 melanoma, 1123 SCC, and 7198 BCC cases were documented. Compared to those whose eye colors were dark or brown, participants with hazel/green/medium and blue/light colors had a 24% (RR = 1.24, 95% CI: 1.06–1.45) and a 19% (RR = 1.19, 95% CI: 1.01–1.41) higher risk of SCC, respectively. Similarly, a higher risk of BCC was observed in participants with hazel/green/medium eye colors (RR = 1.16, 95% CI: 1.09–1.23) and blue/light eye colors (RR = 1.17, 95% CI: 1.10–1.25). We did not find significant associations between eye color and risk of melanoma. Lighter eye color was associated with increased risks of SCC and BCC among those with dark hair colors (p for interaction ≤ 0.02).
In conclusion, in this large prospective study of men, we found that light eye colors were associated with higher risks of SCC and BCC, but not melanoma. Further studies are needed to confirm this association in other populations.
Journal Article